
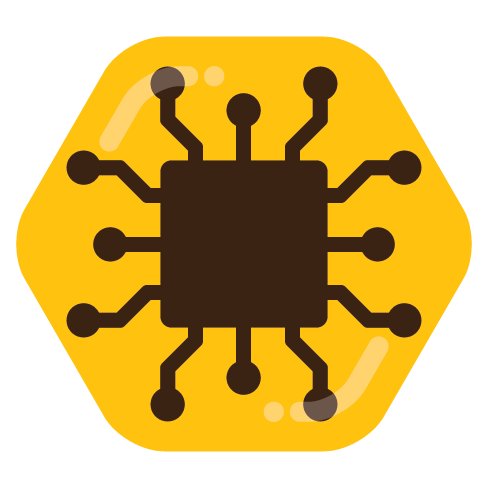
Yeah I have the same with Rollercoaster Tycoon classic. I spent money for it and really enjoyed it, but instead of patching that version for newer phones they just released a “new” version that’s compatible with newer phones. I should’ve expected this from Atari, but damn it’s so scummy
Are you me lol? I did exactly the same, and at some point I started computing how many slides I had to make to make anything larger and I pivoted. My dad didn’t know this stuff but I also ended up at gamemaker